Google ran out of monikers to describe its new AI system released on December 7. Supercomputer perhaps wasn’t an apt description, so it settled on Hypercomputer.
The AI training system is home to Google’s new AI chip, the Cloud TPU v5p, released alongside the Gemini large-language model.
Gemini is Google’s latest attempt to take on Microsoft, which uses GPT-4 to provide AI techniques to answer questions, create documents and images, and much more.
Google timed the release of the TPU V5p chip with the new generative model. The Hypercomputer was also announced to host the new TPU, which is targeted to run the AI workloads.
The system seems to be an example of the hardware-software co-design concept, which all major chip makers are chasing to speed up the time needed to generate AI results. Nvidia typically releases new CUDA releases in conjunction with high-performance GPUs — CUDA 12 was released with the Hopper GPUs.
In a blog entry, Google said its Hypercomputer resulted from “decades of research and development” to develop a system for modern AI workloads. Google claims the chip provides better AI hardware utilization rates, reducing power consumption.
AI applications require vast hardware resources, and cloud providers are developing systems to provide faster results while reducing the cost per transaction. Hyperscalers are investing billions to build data centers loaded with GPUs, AI chips, memory, and high-bandwidth interconnects to process AI queries.
Microsoft has its own AI supercomputer called Eagle, which is now the third-fastest supercomputer in the world, according to the Top500 list. The system delivers 561 petaflops of performance and has 1.12 million computing cores. The horsepower is provided by Nvidia’s H100 GPU and Intel’s Sapphire Rapids CPUs.
Google’s Hypercomputer is built specifically for AI applications, as TPUs aren’t made for scientific computing. The Google system includes a network of pods with Cloud TPU v5p chips. Each pod includes 8,960 chips, which are interconnected via proprietary optical circuit switches (OCS) and provide a throughput of 4,800 Gbps.
The TPU v5p pods are significantly larger in processing scope compared to pods with the older TPUv4 chips, which were introduced in 2020. The TPUv4 pods had 4,096 chips, which are also interconnected via OCS.
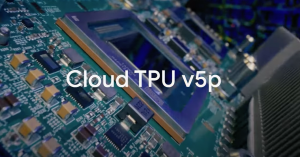
IMAGE – TPU v5p, our most powerful and scalable TPU to date.
“Compared to TPU v4, TPU v5p features more than 2X greater FLOPS and 3X more high-bandwidth memory (HBM),” Google said.
The TPU v5p pods have 95GB of high-bandwidth memory, while the TPU v4 pods have 32GB of HBM. The HBM bandwidth of a TPU v5p pod is 2,765GBps, while the TPU v4 bandwidth was 1228GBps.
The company claimed that TPU v5p can train large-language models 2.8 times faster than TPU v4. However, the data is misleading; the chart showed TPUv5 relative performance measured on the INT8 data type and the TPUv4 based on the BF16 data type.
The TPU v4 did not support INT8, and Google shared a more realistic number — the TPU v5p being 67% faster than TPUv4 on BFloat16.
With TPU v5p, Google now has a chip that can be compared to Nvidia’s H100. Google researchers previously compared the TPUv4 to Nvidia’s A100, in line with the relative product release dates, and claimed the TPU v4 is 1.2x-1.7x faster and used 1.3x-1.9x less power on similarly sized systems.
Earlier this year, Google released another TPU v5 variant, the TPUv5e, which is a power-efficient variant designed for inferencing. Google used TPU v4 and V5e to train the Gemini transformer model. Each TPU v5e chip has four matrix multiplication units, a vector, and a scalar processing unit, all connected to HBM2 memory.
The Hypercomputer with TPU v5p can flip the switch for different types of users and their commitment. The system is flexible enough to assign dedicated resources to big-buck customers with long-term commitments and has more flexible systems for on-demand users. That has helped Google create a more efficient way for customers to consume cloud services.
The consumption system is driven by a feature called the Dynamic Work Scheduler, which is designed so users do not have to wait to execute workloads or see results. Google said the Flex Start mode is “for higher resource obtainability and optimized economics,” and the Calendar mode “targets workloads with higher predictability on job-start times.”
Behind the consumer-facing consumption model is the internal system of software and chips that run the AI models.
The software layer includes an open-source software framework that provides model flexibility with support for JAX, PyTorch, and TensorFlow. Cloud services rely on virtualization technology for provisioning, orchestration, and management of complex AI workloads, which includes slicing up training tasks to virtual machines and coordinating the processing and delivery of results. The Hypercomputer also supports the Google Kubernetes Engine and Compute Engine for container management.
The Hypercomputer software layer also includes support for XLA, which compiles for AI models to run on any hardware. To use AI models, customers can choose between GPUs, CPUs, or Google’s TPUs.
“It optimizes distributed architectures across a wide range of hardware platforms, ensuring easy-to-use and efficient model development for diverse AI use cases,” Google said.
Google already offers supercomputers, such as A3, that hosts 26,000 Nvidia GPUs, but it remains unclear how the Hypercomputer will link up to GPUs. The AI infrastructure typically dedicates specific hardware configurations with forks of generative AI models. Google points to a range of models.
The Hypercomputer is glued together by the Jupiter interconnect, which includes the optical switching system. The 4,800Gbps bandwidth of TPU v5p is two times that of TPUv4.
The optical infrastructure is fitted in a swap-in, swap-out infrastructure that links up AI chips and clusters. Each rack operates independently and can scale up based on computing requirements. The ability to scale computing on the fly and reconfigure the network topology depending on the application and how it fits into Google’s dynamic cloud consumption model.
Google didn’t provide specific details on the number of cores or other specifications in the TPU v5p. But Google introduced many new technologies with the v5e, including Multislice, which can network hundreds of thousands more AI chips together in a cluster. The technology is now supported by TPU v5p.
The TPU v5 first surfaced when Google’s researchers talked about the chip’s development in June 2021. At the time, Google researchers said AI was used to design the chip. The researchers claimed AI floor-planned the chip significantly faster than human experts. Google fired one researcher ahead of the paper’s appearance in Nature magazine, and the company’s claims are now being questioned. It’s unclear if the new chip is based on the paper published by researchers.
Google is making its Hypercomputer available to customers via its cloud service. Pricing is still not available.