- 1CAS Key Laboratory for Plant Diversity and Biogeography of East Asia, Kunming Institute of Botany, Chinese Academy of Sciences, Kunming, Yunnan, China
- 2Key Laboratory of Plant Resources and Biodiversity of Jiangxi Province, Jingdezhen University, Jingdezhen, Jiangxi, China
- 3Germplasm Bank of Wild Species, Kunming Institute of Botany, Chinese Academy of Sciences, Kunming, Yunnan, China
- 4Royal Botanic Garden Edinburgh, Edinburgh, Scotland, United Kingdom
- 5Lijiang Forest Biodiversity National Observation and Research Station, Kunming Institute of Botany, Chinese Academy of Sciences, Lijiang, Yunnan, China
- 6University of Chinese Academy of Sciences, Beijing, China
Selection along environmental gradients may play a vital role in driving adaptive evolution. Nevertheless, genomic variation and genetic adaptation along environmental clines remains largely unknown in plants in alpine ecosystems. To close this knowledge gap, we assayed transcriptomic profiles of late flower bud and early leaf bud of Rhododendron sanguineum var. haemaleum from four different elevational belts between 3,000 m and 3,800 m in the Gaoligong Mountains. By comparing differences in gene expression of these samples, a gene co-expression network (WGCNA) was constructed to identify candidate genes related to elevation. We found that the overall gene expression patterns are organ-specific for the flower and leaf. Differentially expressed unigenes were identified in these organs. In flowers, these were mainly related to terpenoid metabolism (RsHMGR, RsTPS), while in leaves mainly related to anthocyanin biosynthesis (RsCHS, RsF3’5’H). Terpenoids are the main components of flower scent (fragrance) likely attracting insects for pollination. In response to fewer pollinators at higher elevation zone, it seems relatively less scent is produced in flower organs to reduce energy consumption. Secondary metabolites in leaves such as anthocyanins determine the plants’ alternative adaptive strategy to extreme environments, such as selective pressures of insect herbivory from environmental changes and substrate competition in biosynthesis pathways at high elevations. Our findings indicated that the gene expression profiles generated from flower and leaf organs showed parallel expression shifts but with different functionality, suggesting the existence of flexibility in response strategies of plants exposed to heterogeneous environments across elevational gradients. The genes identified here are likely to be involved in the adaptation of the plants to these varying mountainous environments. This study thus contributes to our understanding of the molecular mechanisms of adaptation in response to environmental change.
Introduction
Environmental adaptation and its driving mechanisms in speciation have always been one of the most challenging and unresolved key research aspects in ecology and evolutionary biology (Nelson et al., 2007; Anderson et al., 2011). Adaptation is an evolutionary process in which a species inherits its genetic material by changing its shape or adjusting its survival strategy in order to better adapt to its living environment (Mayr, 1982). In mountain ecosystems, variances in environmental factors due to elevational gradients are considered to be eminently more significant than environmental changes over horizontal gradients. Elevational gradients influence environmental variables, causing major shifts in biotic and abiotic factors within a relatively short geographic distance (Körner, 2007; Liu et al., 2022b), which provides a natural laboratory for the study of environmental adaptation. Compared with low-elevation areas, species inhabiting high-elevation environments are subjected to multiple selective pressure, including low temperature and oxygen levels, high intensity of ultraviolet radiation and strong seasonality (Qiu, 2008; Zhang et al., 2019). Hence, understanding the molecular basis of how species adapt to different environments along elevation gradients can make a significant contribution to our knowledge of adaptation.
Recently, demonstration of how wild species adapt to their local environments has been made easier with the developing of genomic approaches based on next-generation sequencing (NGS) technologies. Especially the transcriptomic analyses have become an effective tool for further research on gene expression, gene regulation, and species adaptive evolution, greatly facilitating eco-evolutionary research on non-model organisms that lack reference genome sequence information (Stapley et al., 2010; Ekblom and Galindo, 2011; Mutz et al., 2013). To date, several studies on the adaptation to divergent alpine environments have been carried out on animals (e.g. Sun et al., 2018; Hao et al., 2019; Qu et al., 2020; Ma et al., 2022a). For plants, a few recent studies have reported the genetic architectures and evolutionary processes driving their adaption along elevational gradients (e.g. Ma et al., 2015; Bohutinska et al., 2021; Liu et al., 2022b). However, intraspecific local adaptation of alpine plants across the extreme elevational gradients existing in the Hengduan Mountains is very scarce, in particular for woody species occurring at an elevation greater than 3,000 m.
Rhododendron (Ericaceae) is a species-rich and ecologically important genus with over 1,000 species globally (Chamberlain et al., 1996), and approximately 590 species occurring in China with a diversification center in the Himalaya-Hengduan Mountains (HHM) (Yan et al., 2015; Fu et al., 2022). Rhododendron underwent rapid adaptive radiation after the Late Miocene in HHM (Mo et al., 2022; Ma et al., 2022b), which led to many sympatrically distributed species in this region (Zou et al., 2021). In addition, Rhododendron can inhabit diverse environments between 1,000 m up to nearly 5,000 m and represent an important component of these heterogeneous ecosystems (Shrestha et al., 2018; Liu et al., 2022b), and environmental heterogeneity was proven to have the highest effects on species diversity in the genus (Shrestha et al., 2018; Xia et al., 2022). Despite increasing awareness of their ecological importance, the molecular mechanisms of Rhododendron species that underpin adaptation to environmental changes along elevation gradients are poorly studied.
Recently, high-throughput RNA Sequencing (RNA-seq) has been widely applied to explore plant responses to high-elevation environments. For example, a combination of transcriptomic and metabolomic analyses revealed that differences in gene expression, evolutionary adaptation rate and metabolites changes are involved in the adaptation of four differently flower-colored Rhododendron species to heterogeneous environments across elevational gradients (Liu et al., 2022b). Rhododendron sanguineum forms a highly color polymorphic complex group including six varieties and is typically located at elevations over 3,000 m, which is associated with snow cover (Fang et al., 2005; Ye et al., 2021). The plants are shrubs that typically grow 30 cm–150 cm in height and are exposed in the open (Fang et al., 2005). Our previous comparative transcriptomics study has clarified the molecular mechanisms of flower color divergence of three sympatrically occurring varieties in this complex (Ye et al., 2021). However, how the R. sanguineum complex has adapted and evolved to the highly heterogenous environments along elevational gradients above 3,000 m remains unclear. The adaptation of plants of one variety, R. sanguineum var. haemaleum, to their specific habitat has likely contributed to its intraspecific diversification, and these plants should be an ideal system for the study of ecological adaptation in a heterogeneous environment.
The present study was designed to reveal adaptation mechanisms at the gene expression level of an alpine Rhododendron sanguineum variety across elevational gradients where the plants occur. We generated gene expression profiles using RNA-seq data from two major organs including late flower and early leaf buds of R. sanguineum var. haemaleum growing at four different elevation zones between 3,000 m and 3,800 m in the Gaoligong Mountains. A comparative transcriptome analysis of the organs from different elevations was performed to determine their gene expression patterns to identify differentially expressed unigenes related to elevation. We then annotated these genes to characterize their likely functions to reveal the response strategies of different organs to heterogenous environments underlying elevation adaptation. This study will provide novel insights into the genetic mechanisms of alpine plant species in response to heterogeneous environments caused by elevation and lay a foundation for further explorations of the genetic changes underlying high elevational adaptation among rhododendrons and other alpine species. In addition, to the best of our knowledge, it is the first comparative transcriptomic study on the adaptation mechanism of an intraspecific alpine woody plant to elevational gradients under field conditions.
Materials and methods
Plant sampling
In June 2018, samples of R. sanguineum var. haemaleum for transcriptome sequencing were collected between 11:00am and 1:30pm within two successive days from four sites along an elevational gradient (Figure 1) in the northern Gaoligong Mountains (N 27°49’53”, E 98°27’03” - N 28°05’13”, E 98°45’31”) which is located in southern of Hengduan Mountains (Liu et al., 2022a). Three individuals were sampled across a small range of 20 m2 from each site. The entire late flower bud and entire early leaf bud were sampled from each individual, immediately frozen in liquid nitrogen in the wild, and preserved in a -80°C ultra-low temperature freezer prior to processing for total RNA isolation. Vouchers of each individual plant sampled were collected and deposited in the Herbarium of Kunming Institute of Botany (KUN), Chinese Academy of Sciences.
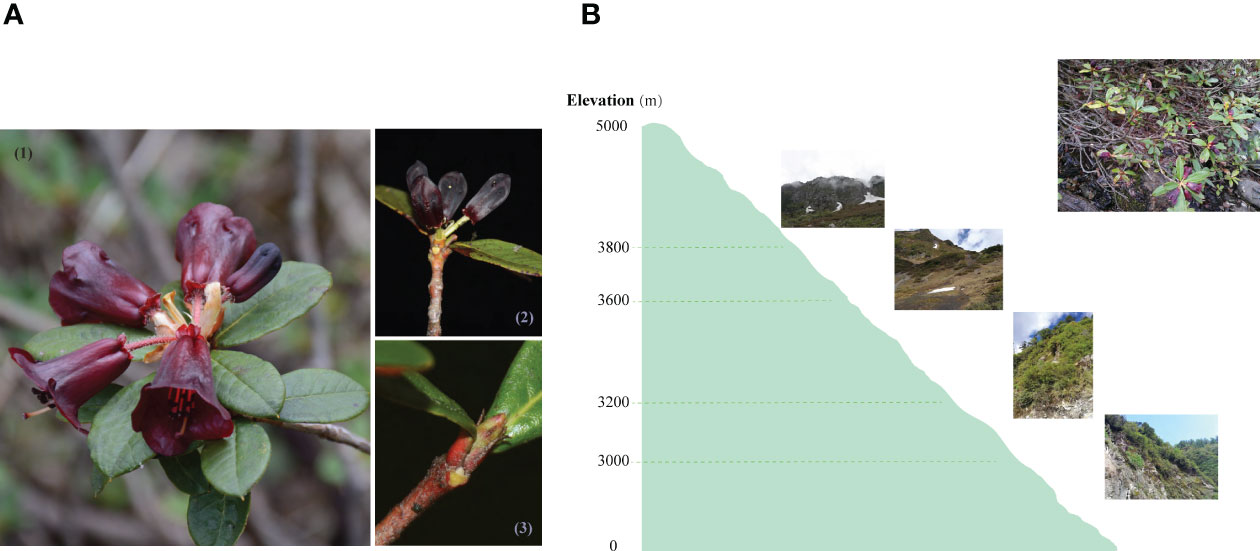
Figure 1 Morphological characters, sampling organs (A), schematic sampling map and wild habitats (B) of R. sanguineum var. haemaleum. Morphology of open flowers and fully expaned leaves (1), sampled stage for entire late flower buds (2), and entire early leaf buds (3).
RNA extraction and transcriptome sequencing
Total RNA was extracted and purified separately from each organ using a Spectrum TM Plant Total RNA Kit (STRN250, Sigma) according to the manufacturer’s protocols. The RNA purity and quality were assessed with a Nanodrop 2000 spectrophotometer (Thermo Fisher Scientific, Waltham, MA, USA). RNA integrity was evaluated through agarose gel electrophoresis. Three biological replicates (from three plants) of each flower bud and leaf bud material were included for each elevational site. A single cDNA library was separately constructed for each flower bud and each leaf bud of each individual from each site, and subsequently sequenced on an Illumina HiSeq X Ten sequencing platform (San Diego, CA, USA), generating approximate 6 Gb paired-end reads (2 × 150 bp) for each sample. Both cDNA library preparation and Illumina sequencing were carried out by Novogene Bioinformatics Technology Co., Ltd. (Beijing, China).
Data processing, De novo assembly and mapping
We first cleaned the raw sequence reads by using Trimmomatic v. 0.38 (Bolger et al., 2014) with default settings to remove low-quality reads. We further evaluated the quality of the remaining reads using FastQC (Andrews, 2010). All subsequent analyses were based on these cleaned reads. A reference transcriptome was generated by de novo assembly of the combined clean reads (leaf buds, flower buds, in three replicates from each gradient) performed using Trinity v. 2.6.5 (Haas et al., 2013) with default parameters. Assembly statistics were obtained using the TrinityStats.pl script in the Trinity package. The longest transcripts were considered as the non-redundant unigenes. We also used HISAT2 v. 2.1.0 (Kim et al., 2019) to assess assembly quality, by mapping reads back to the assembled transcripts to count the overall alignment rates.
Assessment of completeness and gene functional annotation
To lower the redundancy in the dataset, low-coverage artifacts or redundancies were removed using the CD-HIT-EST v. 4.7.0 (Fu et al., 2012) with setting word length to 10 and an identity threshold of 90%. Downstream analyses were performed on the final filtered transcripts. To determine the transcriptome completeness of the assembly, Benchmarking Universal Single-Copy Orthologs tools (BUSCO, v. 4.0.6) (Simao et al., 2015) were used to obtain the percentage of single-copy orthologs represented in the embryophyte database (odb10, 1,614 single-copy orthologues) and also to evaluate the completeness of transcript assemblies. To annotate the assembled unigenes, we downloaded a protein reference of R. delavayi, a species closely related to R. sanguineum from the whole genome sequencing project deposited in GigaDB (Zhang et al., 2017). Open reading frames (ORFs) were first predicted from each filtered assembled transcript using TransDecoder v. 5.5.0 (Haas et al., 2013) with a minimum length of 100 amino acids, and the predicted ORFs were scanned to find homology profiles with a cut-off e-value of 1e-10 against the reference protein database. Unigenes and predicted protein sequences were used as queries to search protein databases using the BLASTP v. 2.5.0, setting the e-value cutoff to 1e-10. Queries were performed against the NCBI non-redundant (NR) and UniProtKB/Swiss-Prot databases. We also performed additional functional annotations with DIAMOND (Buchfink et al., 2015) hits against the eggNOG database (Huerta-Cepas et al., 2017), which summarized available functional information from the different proteins databases, including GO (Gene Ontology), COGs/KOGs (Clusters of Orthologous Groups, containing both prokaryotic and eukaryotic clusters), Pfam (Protein families) and KEGG (Kyoto Encyclopedia of Genes and Genomes) (Hernández-Plaza et al., 2023). The best hit was used as the final annotation.
Transcript abundance and differential expression analyses
To quantify transcript abundance, we applied the alignment-based methods by mapping all of the cleaned reads from the flower bud samples separately for each biological replicate back to the non-redundant unigenes of the assembled reference transcripts using RSEM v. 1.3.1 (Li and Dewey, 2011) and Bowtie2 v. 2.3.5 (Langmead and Salzberg, 2012) for alignment. When the transcript abundance for each biological replicate flower bud sample had been obtained, we generated a gene expression matrix that was constructed from a matrix of read count with a Trinity script. The differentially expressed unigenes (DEUs) analysis were performed with the DESeq2 package (Love et al., 2014) among the four elevation gradients. Samples from the three high elevations were separately compared to those harvested at lower elevation (3,000 m) to construct comparison groups. DEUs were considered those with false discovery rate (FDR) adjusted p values ≤ 0.05 and absolute values of log2 (fold change) ≥ 1. To compare gene expression values across four populations at different elevations, we used the trimmed mean of M-values normalization (TMM), as implemented in the R package edgeR. All downstream analyses were implemented based on the normalized expression data matrix (TMM normalization). The functional enrichment analyses of DEUs from each comparison were further processed as described previously (Ye et al., 2021), including GO (Gene Ontology) and KEGG (Kyoto Encyclopedia of Genes and Genomes). GO terms and metabolic pathways with p values ≤ 0.05 were considered significantly enriched by DEUs.
Construction of weighted gene co-expression network
WGCNA (Weighted gene co-expression network analysis) is an analytical method to explore the relationship between modules and concerned phenotypes and to mine key genes in co-expression networks (Langfelder and Horvath, 2008). To further identify the relevant regulatory gene modules related to elevation, a gene co-expression network was constructed on flower and leaf bud organs, respectively, using the WGCNA package in R (Langfelder and Horvath, 2008). This pipeline included principally three steps. Firstly, a hierarchical cluster analysis was carried out after removing low correlation genes, or those with low expression levels using the hclust function. Secondly, to meet the prerequisite of scale-free network distribution, the soft-power threshold β was determined by the function “sft$powerEstimate” to create an adjacency matrix. To better assess the correlation-based association among gene expression patterns, the adjacency matrix was further converted to a topological overlap matrix (TOM), and a gene connection network was constructed. Finally, gene modules were identified and clustered by a dynamic tree cut method based on the eigengenes (ME) of each module, and the modules with closer distances were merged to obtain the appropriate modules. For the two different organs, the threshold to merge similar modules (mergeCutHeight) and minimal gene module size (minModuleSize) were all set to 0.2 and 100, respectively, except the expression split threshold (deepSplit, flower bud was 2 and leaf bud was 1).
Identification and enrichment analysis of target gene modules
To further investigate the gene modules associated with different environmental conditions, the correlation coefficients between module eigengenes and different altitudes were calculated and statistically tested using Pearson correlation analyses. Modules with absolute values of correlation coefficients > 0.60 (P < 0.01) were considered as altitude-relevant modules: the larger the absolute values of correlation coefficient, the higher the correlation between the module and altitude. Functional enrichment analyses of DEUs in altitude-related modules were carried out by using the AnnotationForge and clusterProfiler package in R (Yu et al., 2012; Carlson and Pages, 2018).
Results
Illumina sequencing, De novo assembly and quality assessment
The transcriptome data of 24 cDNA libraries originating from the two organs (late flower buds and early leaf buds) for three individuals from each of the four elevational samples were obtained in this study. After trimming adapter sequences and removing low-quality sequences, we generated 40,622,598 – 59,403,462 sequence reads with the Q30 percentage of 89.22% – 93.82% and the GC percentage of 46.35% – 48.84% (Supplementary Table S1). A total of ~174.4 Gb clean data was generated after merging all reads of the 24 libraries. The Q30 (99.9% base call accuracy), GC-content of the merged data were 92.60% and 43.52%, respectively (Table 1). All of the clean reads were assembled into transcripts using the de novo assembly tool. In total, 335,121 transcripts were identified with the average length of 1,156 bp and N50 length of 1,707 bp. We selected the longest transcript as the representative for each cluster. All the transcripts were further filtered to obtain 162,995 unigenes with the average length of 907 bp, N50 length of 1,201 bp and GC-content of 42.02% (Table 1). The majority of the assembled unigenes were in the ranges of 300–2,000 bp and 14,698 (9.02%) were over 2,000 bp (Table 1, Supplementary Figure S1B). Assessment of the transcriptome completeness identified 1,543 (95.60%) complete and fragmented BUSCOs based on the 1,614 conserved BUSCO embryophyte orthologs (Supplementary Figure S1A). The reads of the 24 cDNA libraries were aligned by mapping the reads back to the assembled reference transcript, with mapping rates ranging from 93.85% to 96.07% (Supplementary Table S1). These indicated that our transcriptomes were well assembled, and were of good quality.
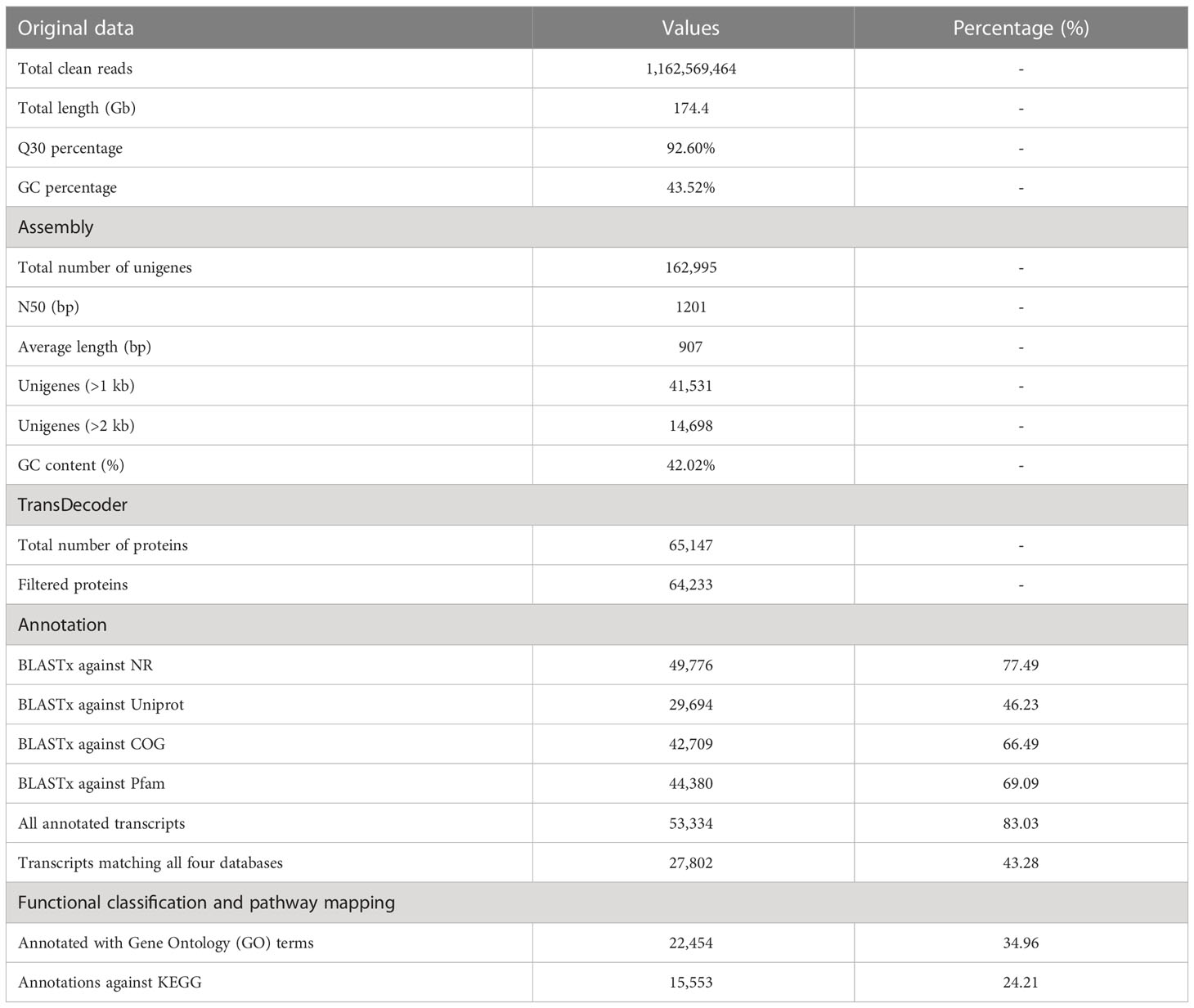
Table 1 Summary of sequencing data, assembly and function annotation of the R. sanguineum var. haemaleum transcriptome.
Functional annotation of the transcriptome
To determine putative functions of the identified unigenes of R. sanguineum var. haemaleum, partial and complete open reading frames (ORFs) were predicted using the TransDecoder software, and CD-HIT-EST was used to cluster protein coding transcripts to obtain the non-redundant proteins. Finally, a total of 64,233 coding proteins were translated from the filtered assembly (Table 1). Similarity searches for each unigene against public databases, including National Center for Biotechnology Information non-redundant (NR) protein database, UniProt, COG and Pfam found 49,7769 (77.49%), 29,694 (46.23%), 42,709 (66.49%), 44,380 (69.09%) matches, respectively. Taken together, 53,334 (83.03% of the total) of the unigenes showed a match in at least one of these searchable databases, and 27,802 (43.28%) showed significant matches in all of the four databases (Table 1; Supplementary Figure S1C). Furthermore, 22,454 (34.96%) unigenes were classified into different GO functional groups. The most abundant GO subcategories for biological processes, molecular functions, and cellular components were cellular process 17,635 (27.45%), catalytic activity 10,098 (15.72%) and cell 19,384 (30.18%), respectively (Table 1; Supplementary Figure S2A). We assigned 15,553 (24.21%) unigenes to 405 KEGG metabolic pathways (Table 1), which represents a valuable resource for investigating specific processes, functions and pathways. Details regarding the KEGG annotation can be found in Supplementary Figure S2B.
Differentially expressed unigenes and functional enrichment
All expression analyses were performed separately for the three biological replicates of the flower and leaf organs. A gene expression matrix with 24 columns and 162,995 lines were generated. Each column represented a sample and each line corresponded to the expression of a unigene. The gene expression density and gene expression distribution of 24 individual libraries in late flower buds and early leaf buds can be found in Supplementary Figure S3. PCA analysis and correlation matrix showed a good correlation between the replicate sets for each elevation of the 24 individual libraries, each elevation and different organ clustered together, showing a population-specific and organ-specific pattern (Figure 2A), which indicated that the RNA-seq libraries were reliable.
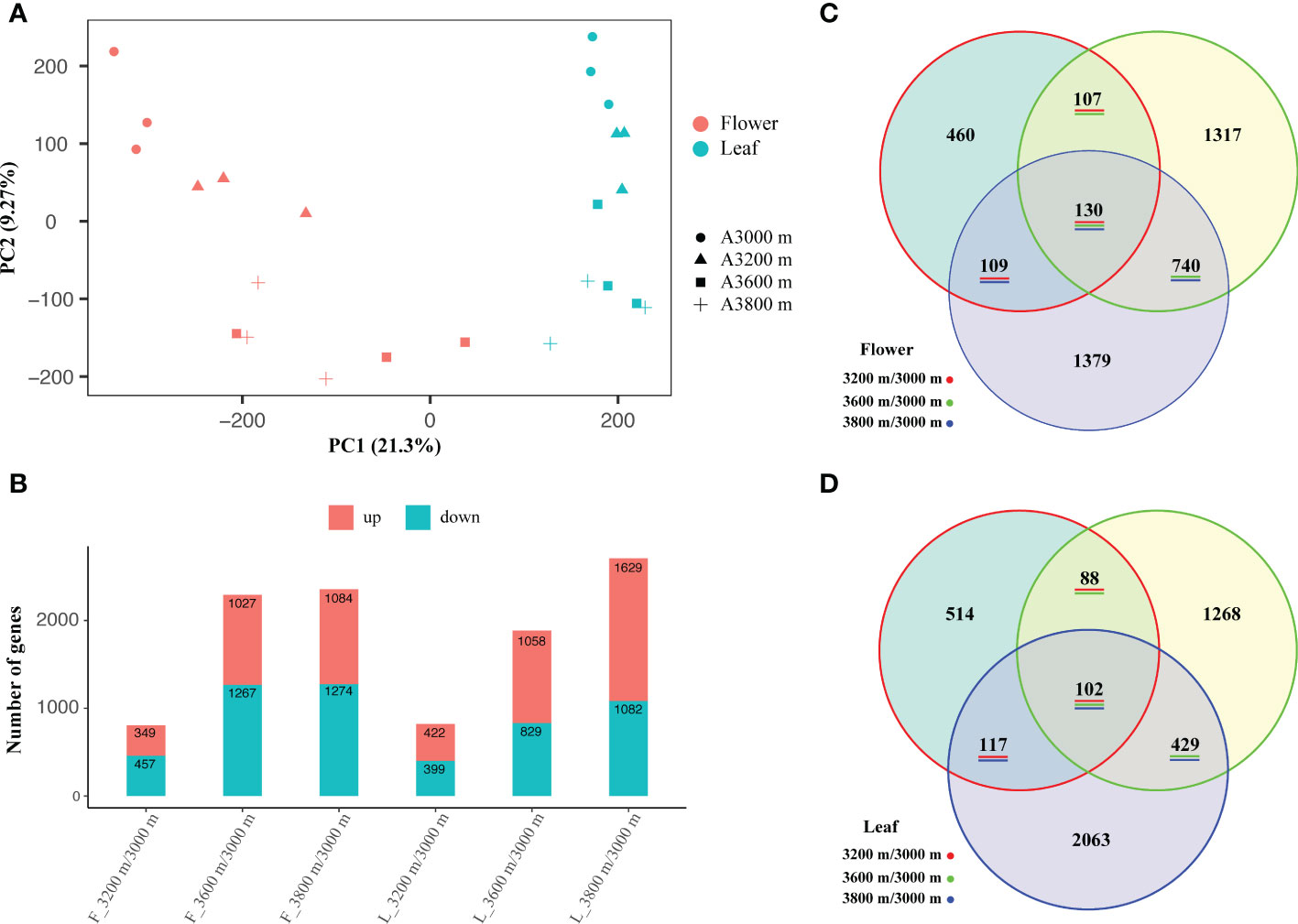
Figure 2 Principal component analysis (PCA) of 24 individuals of the R. sanguineum var. haemaleum (A), and the number statistics (B) and Venn diagrams (C, D) of differentially expressed unigenes (DEUs) among three different comparisons in late flower and early leaf bud, respectively.
Based on the expression profiles at different elevational sites, differentially expressed unigenes (DEUs) were identified by comparing them to the lowest elevation (3,000 m). A total of 2,358 DEUs (up-regulated: 1,084, down-regulated: 1,274) were obtained in the comparison 3,800 m vs. 3,000 m, followed by 3,600 m vs. 3,000 m (2,294, up-regulated: 1,027, down-regulated: 1,267) and 3,200 m vs. 3,000 m (806, up-regulated: 349, down-regulated: 457) in late flower bud, while 2,711 DEUs (up-regulated: 1,629, down-regulated: 1,082), 1,887 DEUs (up-regulated: 1,058, down-regulated: 829), and 821 DEUs (up-regulated: 422, down-regulated: 399) were screened in early leaf bud, respectively (Figure 2B). These graphs indicated that the number of DEUs increased with increasing elevation span in each comparison group of the two organs. In total, we generated 130 DEUs in three comparisons of late flower bud (Figure 2C) and 102 DEUs in early leaf bud (Figure 2D). Details of the DEUs pattern are shown in Supplementary Figure S4.
The GO and KEGG functional enrichment analyses of the shared DEUs in the three comparisons showed that the GO enrichment terms of the DEUs were classified into three categories of gene ontologies: biological processes (BP), cellular components (CC) and molecular functions (MF). As shown in Supplementary Figure S5A, the most significantly enriched GO terms in the two different organs (late flower bud, early leaf bud) were sesquiterpene metabolic processes (GO:0051761) and negative regulation of leaf senescence (GO:1900056), respectively, while the KEGG enrichment analysis showed that the DEUs were significantly enriched in sesquiterpenoid and triterpenoid biosynthesis (ko00909), fat digestion and absorption (ko04975), photosynthesis-antenna proteins (ko00196) and tropane, piperidine and pyridine alkaloid biosynthesis (ko00960) (Supplementary Figure S5B). The genes enriched in these GO terms and KEGG pathways may be closely related to the species’ elevational adaptability.
Weighted gene co-expression network construction and module identification
In the WGCNA analysis, to further explore the specific genes that were highly associated with high-elevation adaptation in different organs, separately performed based on the DEUs from late flower buds and early leaf buds, we independently identified 4,242 and 4,581 DEUs among the three comparisons in the two organs. Based on the scale-free topology criterion (scale-free R2 of 0.80 and mean connectivity close to 0), the soft-power threshold of β =15 and 8 were selected to generate a hierarchical tree of flower and leaf bud, respectively (Figures 3A, B). The gene modules were detected based on the topological overlap matrix (TOM), and genes from the two organs were all assigned into 15 distinct modules based on the similarity of their expression patterns. The numbers of the genes in each module varied greatly from 124 to 880 in late flower bud, and 127 to 759 in early leaf bud, regardless of those unclassified genes in grey modules (Figures 3C, D).
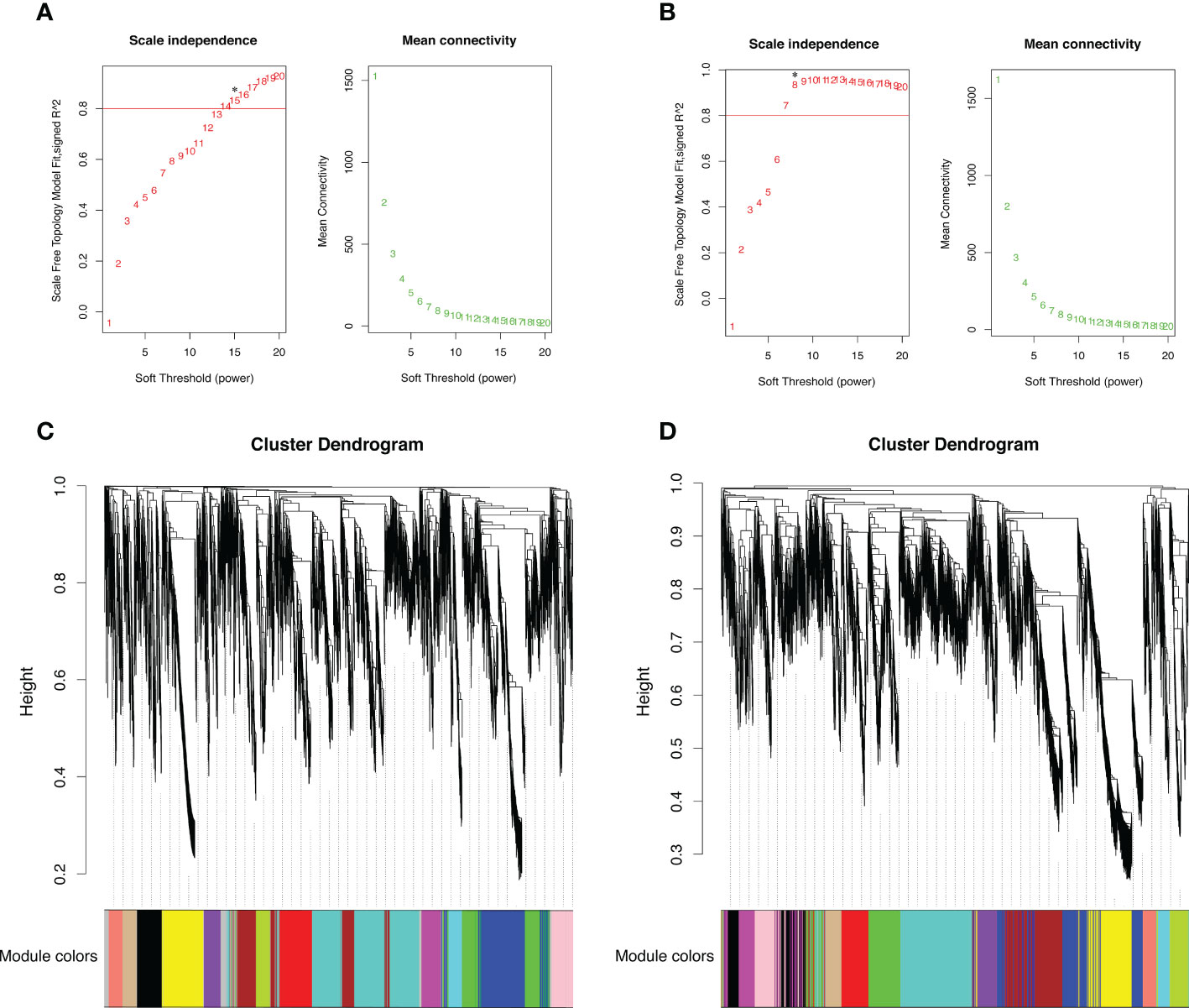
Figure 3 Plot of scale free topology and mean connectivity in regard to soft-thresholding power and Clustering dendrogram showing ortholog expression pattern for samples from (A, C) late flower bud and (B, D) early leaf buds. Red line indicates an R2 cut-off of 0.8. Asterisk indicates the soft threshold power chosen for module detection. Each colored bar below represents each module.
Analysis of elevation related genes in the target modules
To characterize the key modules associated with elevation in R. sanguineum var. haemaleum, we calculated the correlation coefficients between the module and elevation. Modules with the module-trait relationships |r| > 0.60 and p < 0.01 were selected as the key ones that were significantly associated with elevation. Among the 15 gene co-expression modules, four specific modules were obtained in different organs of flowers and leaves, and all of them were significantly negatively correlated with altitude (r < 0), implying that DEUs in these modules may be positively expressed at lower elevations in the flower and leaf organs of R. sanguineum var. haemaleum. The turquoise module was significantly correlated with elevation (r = -0.88, p = 2e-04) (Figure 4), followed by the green module (r = -0.86, p = 3e-04), brown module (r = -0.8, p = 0.002) and red module (r = -0.76, p = 0.004), with 880, 134, 386 and 307 DEUs assigned respectively, in late flower bud. While, in early leaf bud, the tan module (r = -0.89, p = 1e-04) with 171 DEUs, the green module (r = -0.87, p = 3e-04) with 397 DEUs, the red module (r = -0.85, p = 5e-04) with 288 DEUs, and the turquoise module (r = -0.76, p = 0.004) with 759 DEUs were significantly correlated (Figure 4).
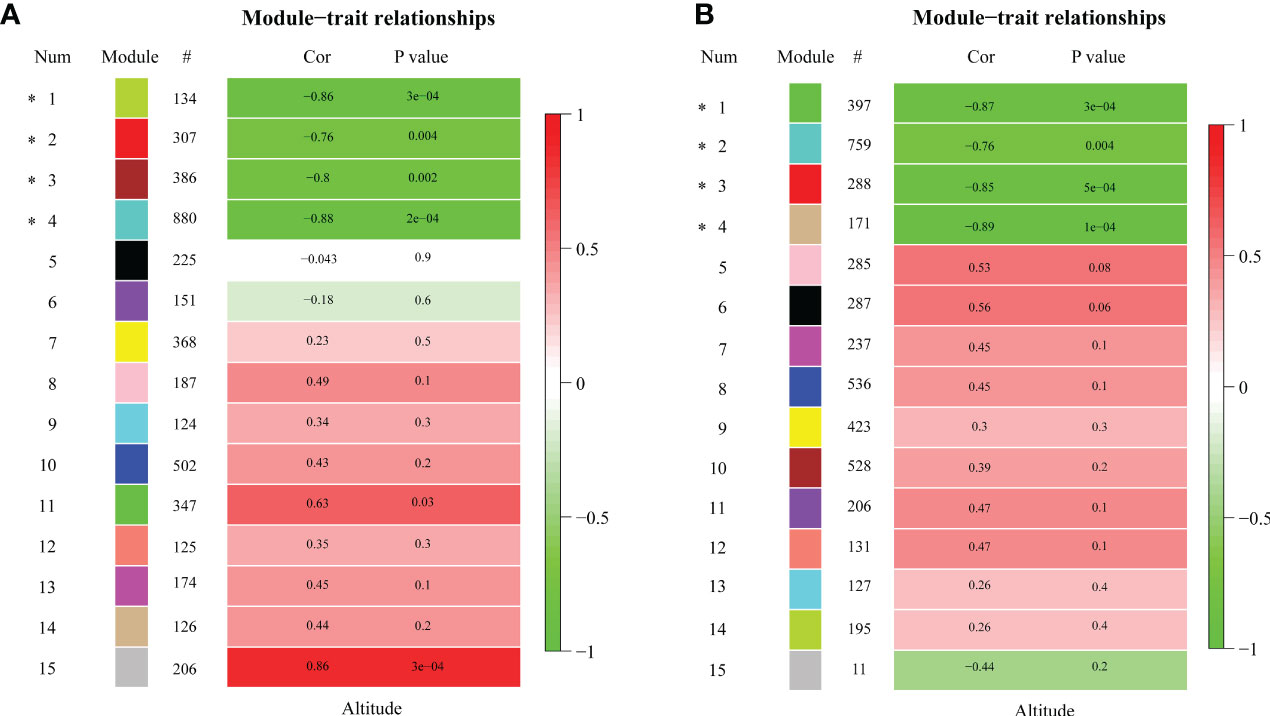
Figure 4 Pearson’s correlation coefficient (Cor) and corresponding significance level (P value) between the expression of genes in each module and the sample altitude from (A) late flower buds and (B) early leaf buds. “#” indicates the number of genes in each module. Asterisk indicates altitude-related module. The color bar to the right of each figure indicates the strength of correlation coefficient.
In the GO and KEGG analyses, conducted to investigate the biological function of the genes in each altitude-related module, the former indicated terpenoid metabolic processes (GO:0006721), response to water deprivation (GO:0009414), plant hormone signal transduction (ko04075) and sesquiterpenoid and triterpenoid biosynthesis (ko00909) as the most significantly enriched functional annotations in late flower buds, and phenylpropanoid biosynthetic processes (GO:0009699), leaf senescence (GO:0010150), flavonoid biosynthesis (ko00941) and photosynthesis-antenna proteins (ko00196) as the most significantly enriched terms/metabolic pathways in early leaf buds. These were the key enrichment objects for the subsequent analysis (Figure 5). Additionally, there were some GO categories or metabolic pathways that were shared in the two organs, while the organ-specific enrichment types were the most striking ones worthy of further analysis, such as sesquiterpene biosynthetic processes (GO:0051762) that occur only in floral organ, and flavonoid biosynthesis (ko00941) unique to leaf organ.
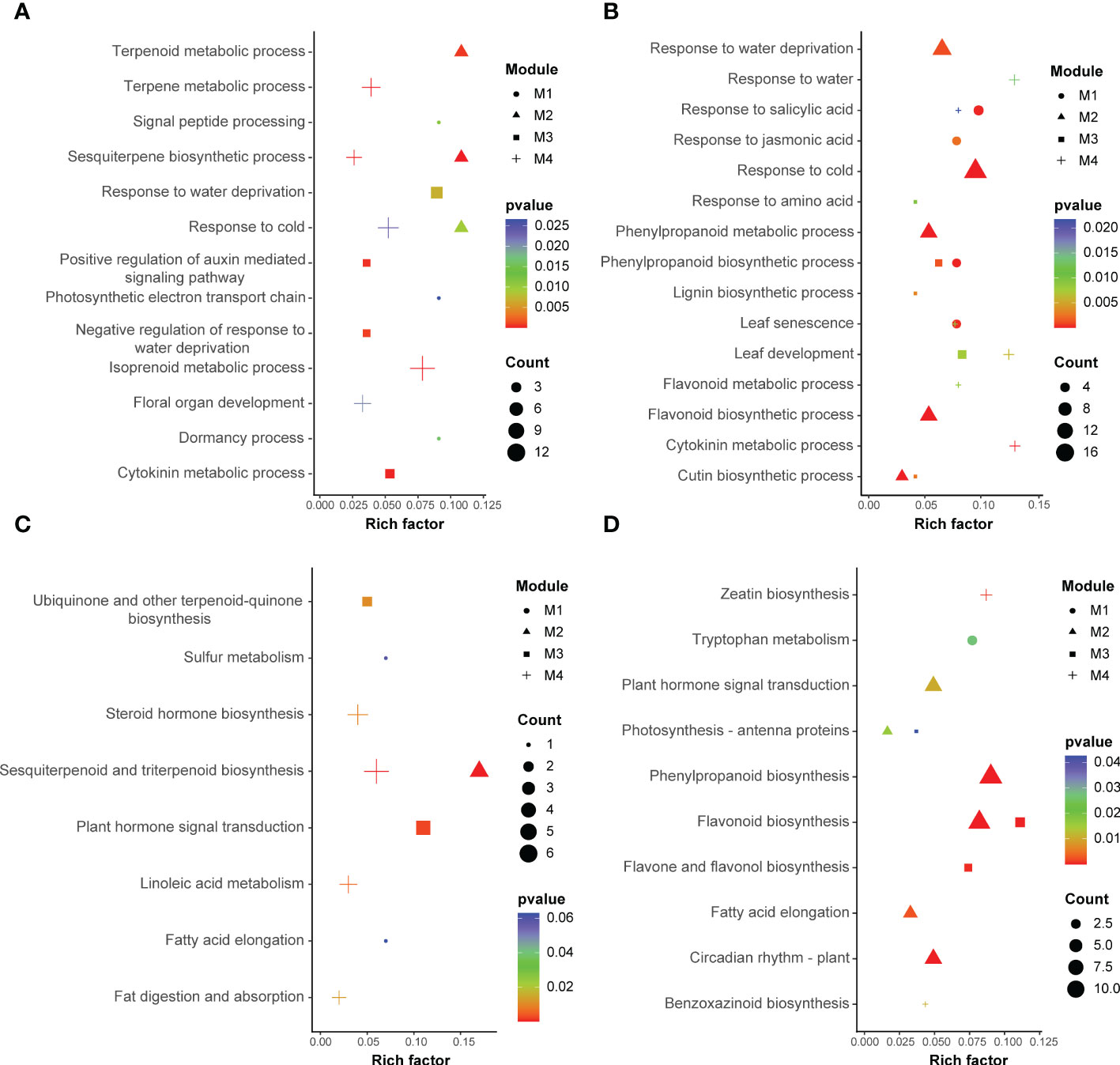
Figure 5 Results of GO enrichment (A, B) and KEGG enrichment (C, D) of significantly expressed unigenes in four different modules identified from late flower buds (A, C) and early leaf buds (B, D), respectively. “M1”, “M2”, “M3” and “M4” refer to the modules that were significantly correlated with altitude.
Based on the result of the GO and KEGG enrichment of DEUs, we confirmed the functional annotations of all candidate genes (Table 2). The sample expression patterns were clustered and visualized in a heatmap to clearly understand the expression of all candidate genes from the two organs (Supplementary Figure S6). In both organs, most gene expression patterns were negatively correlated with altitude, in particular genes related to terpene metabolism in the late flower bud (RsHMGR, RsPT, RsTPS) and anthocyanin metabolism (RsCHS, RsF3’5’H, RsOMT) in the early leaf bud (Table 2). The genes in the terpenoid biosynthesis pathway identified in late flower bud and in the anthocyanin metabolism pathway of early leaf bud were found highly expressed at low elevations and decreased with increasing elevation (Figure 6). Additionally, some genes had multiple homologous copies, with the largest number of 8 and 5 in RsTPS and RsCHS respectively (Table 2), which to a certain extent indicated the importance of these two genes in their respective pathways. However, genes related to each node were not enriched across the entire metabolic pathway (Figure 6).
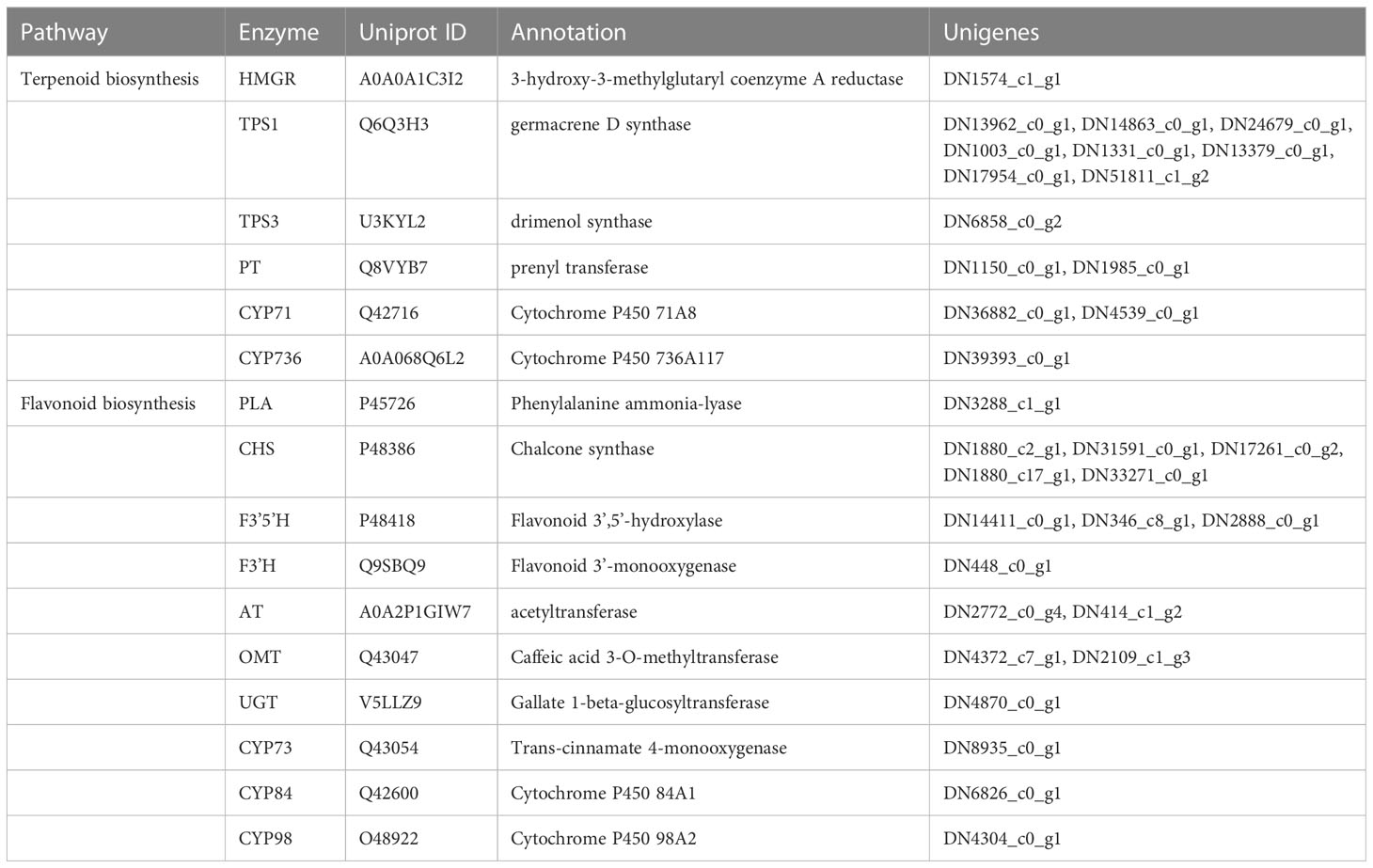
Table 2 Information of the candidate genes associated with elevation, involved in the biosynthetic pathway of late flower bud and early leaf buds.
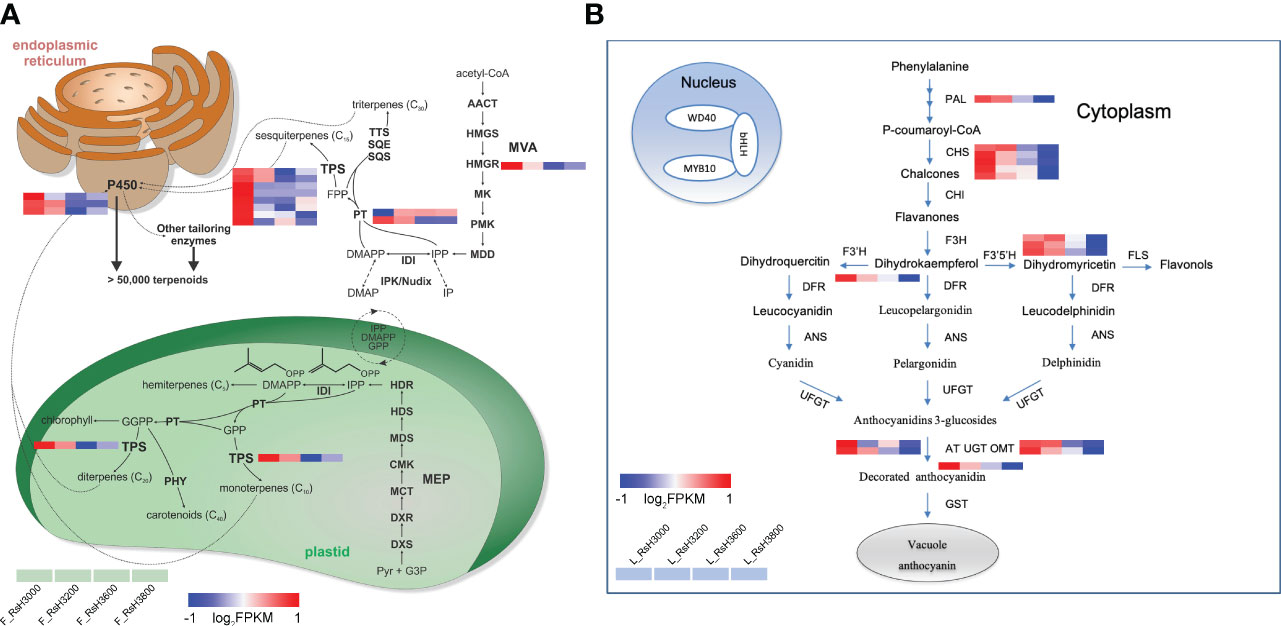
Figure 6 Schematic overview of major terpenoid (A) (modified from Karunanithi and Zerbe, 2019) and flavonoid (B) biosynthetic pathway identified in the organ of late flower buds and early leaf buds, respectively. Altitudes (here RsH3000-RsH3800, from left to right in each heatmap panel) are indicated in the legend. Gene expression profile (in normalized FPKMs) at different elevations are presented in a heatmap alongside the gene names. The bar represents the expression level of each gene. Low to high expression is indicated by a change in color from blue to red, respectively.
Discussion
Gene expression profiles across organs of R. sanguineum var. haemaleum
In this study, we carried out a transcriptome expression profiling analysis in flower and leaf bud sampled along an elevational gradient of R. sanguineum var. haemaleum. The results showed a organ-specific expression profile pattern (Figure 2A), which has been proven in other studies (Ye and Varner, 1991; Leung et al., 2014; Tu et al., 2021). Our results were similar to those of Hao et al. (2019) whose work based on comparative transcriptomics of three closely related bird species at different elevations. The sample replicates, i.e., sampled from three individual plants at each elevation, clustered together but separately for elevation (Figure 2A; Supplementary Figure S6A), indicating that plants growing in the same habitat have a more similar expression profile, while differing from those growing at different elevations. Environmental conditions vary at different elevations, and factors, such as temperature, moisture, light quality, and oxygen, will likely to be involved in determining gene expression levels (Gibson, 2008; Liu et al., 2022b). In addition, by comparing the high and low elevational samples of different organs, i.e., late flower buds and early leaf buds, the number of significantly DEUs in their respective comparisons showed a positive correlation with the magnitude of the elevational distance, i.e., the greater the elevation span, the greater the number of differentially expressed unigenes (Figure 2B). This is consistent with other studies on Potentilla L. (Ma et al., 2015), Bos L. (Ma et al., 2022a) and other species of Rhododendron (Liu et al., 2022b). In this sense, one could hypothesize that along a mountain cline, with increasing elevation, the stresses caused by environmental factors become more severe, and as adaptation of species to these changing environments additional genes are activated, also known as environmental adaptation (Sun et al., 2018; Wang et al., 2021b). Therefore, elevation difference may be the most direct factor in affecting gene expression, and it also indicated that there were no differences between the selected organs (late flower and early leaf bud) on gene expression responses to elevational changes. To some extent, all tissues and organs may cooperate in response to environmental changes.
Gene expression changes accompanying elevational environment adaptation
The results of gene modules related to elevation based on a gene co-expression network analysis (WGCNA) showed negative correlation coefficients between modules and altitude (r < 0) (Figure 4), indicating that genes in these modules were less expressed with higher altitude. We identified different environmental adaptation genes in the two organs. In the late flower bud, they were related to terpenoid metabolism (Table 2; Figures 5A, C), such as RsHMGR (DN1574_c1_g1) and RsTPS (DN13962_c0_g1, DN14863_c0_g1, etc.), which are key genes in terpenoid synthesis, while genes identified only in early leaf bud were mainly related to anthocyanin biosynthesis, leaf senescence and photosynthesis (Table 2; Figures 5B, D), such as the anthocyanin synthesis-related genes RsCHS (DN1880_c2_g1, DN31591_c0_g1, etc.) and RsF3’5’H (DN2888_c0_g1) (Figure 6; Supplementary Figure S6). The expression levels of these genes decreased with increasing elevation. Terpenes are one of three main component classes of flower scent that is one of the most important traits for plant adaptation and evolution (Knudsen et al., 2006; Raguso, 2008; Wang et al., 2021a). Anthocyanins are secondary metabolites belonging to flavonoids determine the resistance of species to extreme environments (Grotewold, 2006; Tanaka et al., 2008). Environmental factors (temperature, light quality, humidity and oxygen concentration) change along an elevational gradient, with temperature and oxygen concentration decreasing with elevation, while light intensity (UV-radiation) increases (Körner, 2007). In Rhododendron plants, scent-related terpenes are the main components of their floral fragrance and the main substances that attract insects (Dudareva et al., 2005; Wang et al., 2021a). Wang et al. (2021a) demonstrated that species in Rhododendron sect. Azaleastrum, mainly distributed at low elevations, have fragrant flowers, but generally exhibit light or faded colors, compared to high-elevation rhododendrons, and the expansion of terpene synthase genes (TPSs) in R. ovatum not only promotes floral scent production but is also related to a greater diversity of the floral fragrant compounds. However, due to the limitation of abiotic factors, pollinators are generally rare in higher elevational environments (Hargreaves et al., 2015; Tong et al., 2021). Although the successful attraction of plant floral organs to pollinators determines the reproductive success rate of a species, to a certain extent, there must be an evolutionary trade-off for plants to adapt to the environment in this long evolutionary process (Armbruster, 2014). Because more fragrance production equates to more energy consumption, while it is extremely difficult for plant biomass accumulation where induced by harsh environmental factors at high elevations (Lavorel and Grigulis, 2012), when it is only to attract insects that are relatively rare there (Totland, 2001). It is also a balance for pay (energy expenditure on floral fragrance) and gain (attraction of pollinators). Notably, our study supported the hypothesis of a contrasting living strategy that were proposed for Trifolium repens (Hofmann and Jahufer, 2011) and Cyclocarya paliurus (Du et al., 2021). This is also a manifestation of plant adaptation to the environment as a long evolutionary process (Cruden, 1972; Hargreaves et al., 2015).
The accumulated anthocyanins are secondary metabolites of flavonoids (Tanaka et al., 2008; Jaakola, 2013), usually implicated in flower color as in the variety here (Ye et al., 2021). They are also frequently produced in young shoots, including leaf buds (Lee, 2002). Leaf buds often flush red synchronously during expansion that can be a widespread and visually striking phenomenon (Karageorgou and Manetas, 2006; Alberto et al., 2011). However, the red flushing appearance of leaf buds (as indicated in Figure 1A (3)) in many plants is transient, and most leaves contain considerable quantities of anthocyanins only in the juvenile stages (Dominy et al., 2002; Zhang et al., 2016), and can be a response to environmental stress (Lee, 2002). Among various environmental factors that affect anthocyanin biosynthesis, light intensity (UV radiation) and temperature are particularly important (Jaakola and Hohtola, 2010; Naing and Kim, 2021). It is generally acknowledged that strong light intensity can enhance the expression of genes related to anthocyanin biosynthesis and increase anthocyanin levels (Jaakola, 2013; Zhang et al., 2018). Studies have shown that within a certain range, light intensity can activate phytochromes and promote the synthesis or activation of a series of light-regulated enzymes such as phenylalanine lyase (PAL), chalcone synthase (CHS), and flavonoid glucosidase (UFGT), which affect the content and proportion of anthocyanins, thereby resulting in the coloration of leaves (Jaakola and Hohtola, 2010; Dong and Lin, 2021). However, the response of plants to light intensity varies in different environmental conditions. For example, Senica et al. (2017) revealed an increased anthocyanin content with increasing elevation in leaves of elderberry (Sambucus nigra), and Du et al. (2021) for Cyclocarya paliurus. Therefore, we hypothesize that increase on anthocyanin levels may be linked to the increase in light intensity across a certain elevational range. While at extremely high-elevation environments (> 3,000 m), gene expression is inhibited and hinders the synthesis and accumulation of anthocyanin in an early leaf bud. It has been shown that foliar anthocyanins are correlated with resistance to biotic and abiotic agents such as herbivores, cold and excess radiation (Gould et al., 2000; Close and Beadle, 2003). On the one hand, observations in the field showed that leaf buds, especially at lower elevation may be susceptible to herbivore damage, for insects may show preferences for young leaves for food or oviposition (Close and Beadle, 2003). Therefore, selective pressures of insect herbivory at lower elevations could elicit increased anthocyanin production (antiherbivore hypothesis) (Kost et al., 2020), because foliar anthocyanins and some other phenolics can serve as potent deterrents against generalist herbivores (Karageorgou and Manetas, 2006; Kost et al., 2020). On the other hand, excessive light intensities at high elevations may predispose leaves to photoinhibition of photosynthesis and may also cause damage to leaf buds because of their immature photosynthetic machinery (Miranda et al., 1981). However, Karageorgou and Manetas (2006) found that leaf thickness rather than anthocyanins could appreciably reduce the risk of photo-oxidative damage in young leaves in the field, and even the photoprotective function of anthocyanin accumulations in mature leaves under stress is transient. Thus, the effect of leaf thickness on photoprotection effectiveness may have been underestimated, which generally happened at high elevation. It could thus also be hypothesized that a substrate competition exists between lignin and anthocyanin biosynthesis (Hu et al., 2021). Plants occurring in high elevations can increase lignin deposits and thickness in the cell walls by competing for common substrates under UV exposure to reduce potential excessive light damage (Kost et al., 2020). In the present study, the down-regulation of DEUs involved in the anthocyanin metabolic pathway indicates that R. sanguineum var. haemaleum at high elevation experience a reduction in the expression of genes related to anthocyanin accumulation. This reduction is possibly induced by selective pressures of insect herbivory from environmental changes and substrate competition in the biosynthesis pathway. Our results are in accordance with a study on Zea mays where lowland maize landraces showed higher expression of several confirmed and putative genes involved in anthocyanin biosynthesis as compared to highland landraces (Kost et al., 2020). In addition, a high light intensity is also accompanied by low temperatures in high elevational environments, and studies have shown that low temperatures inhibit anthocyanin synthesis (Christie et al., 1994; Naing and Kim, 2021). Choi et al. (2009) reported that anthocyanin accumulation is light dependent at low temperatures and its synthesis is down-regulated at low temperatures. Collectively, the low expression level of anthocyanin biosynthesis-related genes at higher elevations can therefore be ascribed to a combined effect of biotic and abiotic factors.
Response strategies of flowers and leaves to high elevational environments
The adaptation of organisms to elevational environments is a complex process (Abbott and Brennan, 2014; Sun et al., 2014). In plant species, some response mechanisms and related signalling systems are excited and reinforced to adapt to different environmental stresses (Weigel and Nordborg, 2015; Hao et al., 2019). As the two most important organs of plants, those of flowers (as reproductive organs) and leaves (as primary photosynthetic organs), representing the above-ground parts of the plant, their occurrence along an elevational gradient present, to a certain extent, the species’ responsiveness to environmental variation (Frei et al., 2014; Lopez-Goldar and Agrawal, 2021). In the present study, we found that the metabolic pathways and candidate genes related to altitude identified from flowers and leaves were significantly different (Figure 5; Table 2). Genes from the late flower bud were mainly related to terpenoid metabolism, fatty acid elongation and flower organ development, while genes related to anthocyanin biosynthesis, photosynthetic antenna proteins and leaf senescence were identified in early leaf buds. Moreover, the expression of these genes was negatively correlated with altitude, that is, the higher the altitude, the lower their expression levels. When plants experience abiotic stress, they try to adapt to the stressful condition by adjusting the expression of a series of genes involved in complex networks (Nagano et al., 2019; Geng et al., 2021; Tokunaga et al., 2022).
Studies have shown that flower organ, as a very short-lived reproductive organ, mainly functions to attract pollinators, and produce seeds, while leaf organ is responsible for photosynthesis and energy supply (Bazzaz et al., 1979; Alderson and Rowland, 1995), and are long-lived in the evergreen Rhododendron sanguineum. Thus, it is not surprising that these two organ types behave very differently in response to changes in elevation. Terpenoids are the largest class of secondary metabolites in plants (Dudareva et al., 2005). Terpenes can be emitted from vegetative and floral organs to protect organs from heat burns produced by photosynthesis, and they can also participate in certain chemical ecological processes as signal molecules to attract insects and other pollinators (Pichersky and Gershenzon, 2002; Dudareva et al., 2004). Anthocyanins are secondary metabolites of flavonoids and play an important role in plant stress response (Tanaka et al., 2008; Du et al., 2021). The leaf is the main organ of plants exposed to air for photosynthesis, and it is more sensitive to the surrounding environment (including biotic and abiotic factors), mainly in the content of secondary metabolites (Hartmann, 1996; Landi et al., 2020). Moreover, under the long-term influence of external ecological factors, the morphological structure of leaves has great variability and plasticity, which is generally closely related to plant growth strategies and the ability to utilize resources (Obeso, 2002; Taylor et al., 2012; Rathee et al., 2021). Consequently, there are obvious functional divisions and constraints between the two organs from an ecological point of view.
Conclusion
In the present study, comparative analysis of transcriptome data on late flower and early leaf bud at four different elevations of R. sanguineum var. haemaleum showed that the overall gene expression patterns clustered independently according to the different organs used. Different environmental adaptation genes identified in the floral and leaf organs are mainly related to terpenoid metabolism (RsHMGR, RsTPS) and anthocyanin biosynthesis (RsCHS, RsF3’5’H), respectively. The expression levels of these genes decreased with increasing elevation, which also determined the type of secondary metabolite to some extent. The heterogeneous environment resulting from the elevational change may be the main factor affecting gene expression, which illustrated that plant species may adopt varying adaptive strategies to cope with environmental stresses. However, the response of plants to the environment is a complex and comprehensive process, and it is also the result of the coordination and interaction of various traits. Thus, further study on the determinants of secondary metabolite content and quantification of environmental variables across elevations should be investigated to precisely elucidate the plant’s adaptability. Overall, this study provides new insights into the molecular mechanisms of an alpine species’ response to heterogeneous environment caused by elevation, and will shed insights into further studies on high elevational adaptation among rhododendrons and other alpine species in general.
Data availability statement
The data presented in the study are deposited in the National Center for Biotechnology Information (NCBI) Sequence Read Archive (SRA) repository. The names of the repository/repositories and accession number(s) can be found below: http://www.ncbi.nlm.nih.gov/bioproject/PRJNA916369.
Author contributions
LG and DL conceived and designed the research. LY, YL, JZ and WZ carried out field work and sample collection. LY performed the experiments and analyzed the transcriptomic data with assistance from JZ. LY wrote the original draft with critical input from MM and LG. All authors contributed to the article and approved the submitted version.
Funding
This study was supported by the National Natural Science Foundation of China (91631101, 31670213, 32260149, 31970363), the Strategic Priority Research Program of Chinese Academy of Sciences (XDB31000000), the Key Basic Research program of Yunnan Province, China (202101BC070003), and the International Partnership Program of Chinese Academy of Sciences (151853KYSB20190027). RBGE is supported by the Scottish Government’s Rural and Environmental Science and Analytical Services Division.
Acknowledgments
The authors are grateful to Dr. Kanae Nishii (Kanagawa University and Royal Botanic Garden Edinburgh, RBGE) for insightful comments on an early version of this manuscript. Laboratory work and data analyses were performed at the Laboratory of Molecular Biology and iFlora High Performance Computing Center of the Germplasm Bank of Wild Species, Kunming Institute of Botany, Chinese Academy of Sciences.
Conflict of interest
The authors declare that the research was conducted in the absence of any commercial or financial relationships that could be construed as a potential conflict of interest.
The reviewer JG declared a shared affiliation with the authors LY, YL, JZ, WZ, JL, DL, LG to the handling editor at the time of review.
Publisher’s note
All claims expressed in this article are solely those of the authors and do not necessarily represent those of their affiliated organizations, or those of the publisher, the editors and the reviewers. Any product that may be evaluated in this article, or claim that may be made by its manufacturer, is not guaranteed or endorsed by the publisher.
Supplementary material
The Supplementary Material for this article can be found online at: https://www.frontiersin.org/articles/10.3389/fpls.2023.1133065/full#supplementary-material
References
Abbott, R. J., Brennan, A. C. (2014). Altitudinal gradients, plant hybrid zones and evolutionary novelty. Phil. Trans. R. Soc. B. 369 (1648), 20130346. doi: 10.1098/rstb.2013.0346
Alberto, F., Bouffier, L., Louvet, J. M., Lamy, J. B., Delzon, S., Kremer, A. (2011). Adaptive responses for seed and leaf phenology in natural populations of sessile oak along an altitudinal gradient. J. Evol. Biol. 24 (7), 1442–1454. doi: 10.1111/j.1420-9101.2011.02277.x
Alderson, P., Rowland, M. (1995). “Flowering plants,” in Making use of biology. Eds. Alderson, P., Rowland., M. (London: Macmillan Education UK), 117–128.
Anderson, J. T., Willis, J. H., Mitchell-Olds, T. (2011). Evolutionary genetics of plant adaptation. Trends Genet. 27 (7), 258–266. doi: 10.1016/j.tig.2011.04.001
Andrews, S. (2010) FastQC: a quality control tool for high throughput sequence data. Available at: http://www.bioinformatics.babraham.ac.uk/projects/fastqc).
Armbruster, W. S. (2014). Floral specialization and angiosperm diversity: Phenotypic divergence, fitness trade-offs and realized pollination accuracy. AoB Plants 6, plu003. doi: 10.1093/aobpla/plu003
Bazzaz, F. A., Carlson, R. W., Harper, J. L. (1979). Contribution to reproductive effort by photosynthesis of flowers and fruits. Nature 279 (5713), 554–555. doi: 10.1038/279554a0
Bohutinska, M., Vlcek, J., Yair, S., Laenen, B., Konecna, V., Fracassetti, M., et al. (2021). Genomic basis of parallel adaptation varies with divergence in Arabidopsis and its relatives. Proc. Natl. Acad. Sci. U.S.A. 118 (21), e2022713118. doi: 10.1073/pnas.2022713118
Bolger, A. M., Lohse, M., Usadel, B. (2014). Trimmomatic: A flexible trimmer for illumina sequence data. Bioinformatics 30 (15), 2114–2120. doi: 10.1093/bioinformatics/btu170
Buchfink, B., Xie, C., Huson, D. H. (2015). Fast and sensitive protein alignment using DIAMOND. Nat. Methods 12 (1), 59–60. doi: 10.1038/nmeth.3176
Carlson, M., Pages, H. (2018). AnnotationForge: Code for building annotation database packages. R package version 1.24.0. doi: 10.18129/B9.bioc.AnnotationForge
Chamberlain, D., Hyam, R., Argent, G., Fairweather, G., Walter, K. S. (1996). The genus rhododendron: Its classification and synonymy (Edinburgh: Royal Botanic Garden Edinburgh).
Choi, S., Kwon, Y. R., Hossain, M. A., Hong, S. W., Lee, B. H., Lee, H. (2009). A mutation in ELA1, an age-dependent negative regulator of PAP1/MYB75, causes UV- and cold stress-tolerance in Arabidopsis thaliana seedlings. Plant Sci. 176 (5), 678–686. doi: 10.1016/j.plantsci.2009.02.010
Christie, P. J., Alfenito, M. R., Walbot, V. (1994). Impact of low-temperature stress on general phenylpropanoid and anthocyanin pathways: Enhancement of transcript abundance and anthocyanin pigmentation in maize seedlings. Planta 194 (4), 541–549. doi: 10.1007/BF00714468
Close, D. C., Beadle, C. L. (2003). The ecophysiology of foliar anthocyanin. Bot. Rev. 69 (2), 149–161. doi: 10.1663/0006-8101(2003)069[0149:TEOFA]2.0.CO;2
Cruden, R. W. (1972). Pollinators in high-elevation ecosystems: relative effectiveness of birds and bees. Science 176 (4042), 1439–1440. doi: 10.1126/science.176.4042.1439
Dominy, N. J., Lucas, P. W., Ramsden, L. W., Riba-Hernandez, P., Stoner, K. E., Turner, I. M. (2002). Why are young leaves red? Oikos 98 (1), 163–176. doi: 10.1034/j.1600-0706.2002.980117.x
Dong, N. Q., Lin, H. X. (2021). Contribution of phenylpropanoid metabolism to plant development and plant-environment interactions. J. Integr. Plant Biol. 63 (1), 180–209. doi: 10.1111/jipb.13054
Du, Z., Lin, W., Yu, B., Zhu, J., Li, J. (2021). Integrated metabolomic and transcriptomic analysis of the flavonoid accumulation in the leaves of Cyclocarya paliurus at different altitudes. Front. Plant Sci. 12. doi: 10.3389/fpls.2021.794137
Dudareva, N., Andersson, S., Orlova, I., Gatto, N., Reichelt, M., Rhodes, D., et al. (2005). The nonmevalonate pathway supports both monoterpene and sesquiterpene formation in snapdragon flowers. Proc. Natl. Acad. Sci. U.S.A. 102 (3), 933–938. doi: 10.1073/pnas.0407360102
Dudareva, N., Pichersky, E., Gershenzon, J. (2004). Biochemistry of plant volatiles. Plant Physiol. 135 (4), 1893–1902. doi: 10.1104/pp.104.049981
Ekblom, R., Galindo, J. (2011). Applications of next generation sequencing in molecular ecology of non-model organisms. Heredity (Edinb) 107 (1), 1–15. doi: 10.1038/hdy.2010.152
Fang, M. Y., Fang, R. C., He, M. Y., Hu, L. C., Yang, H. P., Chamberlain, D. F. (2005). “Rhododendron,” in Flora of China vol. 14. Eds. Wu, Z. Y., Raven, P. H. (Beijing and Missouri Botanical Garden, St. Louis: Science Press).
Frei, E. R., Ghazoul, J., Matter, P., Heggli, M., Pluess, A. R. (2014). Plant population differentiation and climate change: responses of grassland species along an elevational gradient. Global Change Biol. 20 (2), 441–455. doi: 10.1111/gcb.12403
Fu, C. N., Mo, Z. Q., Yang, J. B., Cai, J., Ye, L. J., Zou, J. Y., et al. (2022). Testing genome skimming for species discrimination in the large and taxonomically difficult genus Rhododendron. Mol. Ecol. Resour. 22 (1), 404–414. doi: 10.1111/1755-0998.13479
Fu, L. M., Niu, B. F., Zhu, Z. W., Wu, S. T., Li, W. Z. (2012). CD-HIT: Accelerated for clustering the next-generation sequencing data. Bioinformatics 28 (23), 3150–3152. doi: 10.1093/bioinformatics/bts565
Geng, Y., Guan, Y., Lu, S., An, M., Crabbe, M. J. C., Qi, J., et al. (2021). Genomic analysis of field pennycress (Thlaspi arvense) provides insights into mechanisms of adaptation to high elevation. BMC Biol. 19 (1), 143. doi: 10.1186/s12915-021-01079-0
Gibson, G. (2008). The environmental contribution to gene expression profiles. Nat. Rev. Genet. 9 (8), 575–581. doi: 10.1038/nrg2383
Gould, K. S., Markham, K. R., Smith, R. H., Goris, J. J. (2000). Functional role of anthocyanins in the leaves of Quintinia serrata a. cunn. J. Exp. Bot. 51 (347), 1107–1115. doi: 10.1093/jexbot/51.347.1107
Grotewold, E. (2006). The genetics and biochemistry of floral pigments. Annu. Rev. Plant Biol. 57, 761–780. doi: 10.1146/annurev.arplant.57.032905.105248
Haas, B. J., Papanicolaou, A., Yassour, M., Grabherr, M., Blood, P. D., Bowden, J., et al. (2013). De novo transcript sequence reconstruction from RNA-seq using the trinity platform for reference generation and analysis. Nat. Protoc. 8 (8), 1494–1512. doi: 10.1038/nprot.2013.084
Hao, Y., Xiong, Y., Cheng, Y., Song, G., Jia, C., Qu, Y., et al. (2019). Comparative transcriptomics of 3 high-altitude passerine birds and their low-altitude relatives. Proc. Natl. Acad. Sci. U.S.A. 116 (24), 11851–11856. doi: 10.1073/pnas.1819657116
Hargreaves, A. L., Weiner, J. L., Eckert, C. G., Bartomeus, I. (2015). High-elevation range limit of an annual herb is neither caused nor reinforced by declining pollinator service. J. Ecol. 103 (3), 572–584. doi: 10.1111/1365-2745.12377
Hartmann, T. (1996). “Diversity and variability of plant secondary metabolism: A mechanistic view,” in Proceedings of the 9th international symposium on insect-plant relationships. Eds. Städler, E., Rowell-Rahier, M., Bauer., R. (Dordrecht: Springer Netherlands).
Hernández-Plaza, A., Szklarczyk, D., Botas, J., Cantalapiedra, Carlos, P., Giner-Lamia, J., et al. (2023). eggNOG 6.0: enabling comparative genomics across 12535 organisms. Nucleic Acids Res. 51 (D1), D389–D394. doi: 10.1093/nar/gkac1022
Hofmann, R. W., Jahufer, M. Z. (2011). Tradeoff between biomass and flavonoid accumulation in white clover reflects contrasting plant strategies. PloS One 6 (4), e18949. doi: 10.1371/journal.pone.0018949
Hu, Y., Cheng, H., Zhang, Y., Zhang, J., Niu, S., Wang, X., et al. (2021). The MdMYB16/MdMYB1-miR7125-MdCCR module regulates the homeostasis between anthocyanin and lignin biosynthesis during light induction in apple. New Phytol. 231 (3), 1105–1122. doi: 10.1111/nph.17431
Huerta-Cepas, J., Forslund, K., Coelho, L. P., Szklarczyk, D., Jensen, L. J., Von Mering, C., et al. (2017). Fast genome-wide functional annotation through orthology assignment by eggNOG-mapper. Mol. Biol. Evol. 34 (8), 2115–2122. doi: 10.1093/molbev/msx148
Jaakola, L. (2013). New insights into the regulation of anthocyanin biosynthesis in fruits. Trends Plant Sci. 18 (9), 477–483. doi: 10.1016/j.tplants.2013.06.003
Jaakola, L., Hohtola, A. (2010). Effect of latitude on flavonoid biosynthesis in plants. Plant Cell Environ. 33 (8), 1239–1247. doi: 10.1111/j.1365-3040.2010.02154.x
Karageorgou, P., Manetas, Y. (2006). The importance of being red when young: anthocyanins and the protection of young leaves of Quercus coccifera from insect herbivory and excess light. Tree Physiol. 26 (5), 613–621. doi: 10.1093/treephys/26.5.613
Karunanithi, P. S., Zerbe, P. (2019). Terpene synthases as metabolic gatekeepers in the evolution of plant terpenoid chemical diversity. Front. Plant Sci. 10. doi: 10.3389/fpls.2019.01166
Kim, D., Paggi, J. M., Park, C., Bennett, C., Salzberg, S. L. (2019). Graph-based genome alignment and genotyping with HISAT2 and HISAT-genotype. Nat. Biotechnol. 37 (8), 907–915. doi: 10.1038/s41587-019-0201-4
Knudsen, J. T., Eriksson, R., Gershenzon, J., Ståhl, B. (2006). Diversity and distribution of floral scent. Bot. Rev. 72 (1), 1–120. doi: 10.1663/0006-8101(2006)72[1:Dadofs]2.0.Co;2
Körner, C. (2007). The use of a’ltitude’ in ecological research. Trends Ecol. Evol. 22 (11), 569–574. doi: 10.1016/j.tree.2007.09.006
Kost, M. A., Perales, H., Wijeratne, S., Wijeratne, A. J., Stockinger, E. J., Mercer, K. L. (2020). Transcriptional differentiation of UV-b protectant genes in maize landraces spanning an elevational gradient in chiapas, Mexico. Evol. Appl. 13 (8), 1949–1967. doi: 10.1111/eva.12954
Landi, M., Zivcak, M., Sytar, O., Brestic, M., Allakhverdiev, S. I. (2020). Plasticity of photosynthetic processes and the accumulation of secondary metabolites in plants in response to monochromatic light environments: A review. Biochim. Biophys. Acta Bioenerg. 1861 (2), 148131. doi: 10.1016/j.bbabio.2019.148131
Langfelder, P., Horvath, S. (2008). WGCNA: an r package for weighted correlation network analysis. BMC Bioinf. 9, 559. doi: 10.1186/1471-2105-9-559
Langmead, B., Salzberg, S. L. (2012). Fast gapped-read alignment with bowtie 2. Nat. Methods 9 (4), 357–359. doi: 10.1038/nmeth.1923
Lavorel, S., Grigulis, K. (2012). How fundamental plant functional trait relationships scale-up to trade-offs and synergies in ecosystem services. J. Ecol. 100 (1), 128–140. doi: 10.1111/j.1365-2745.2011.01914.x
Lee, D. W. (2002). Anthocyanins in leaves: Distribution, phylogeny and development. Adv. Bot. Res. 37, 37–53. doi: 10.1016/S0065-2296(02)37042-3
Leung, P. T. Y., Ip, J. C. H., Mak, S. S. T., Qiu, J. W., Lam, P. K. S., Wong, C. K. C., et al. (2014). De novo transcriptome analysis of Perna viridis highlights tissue-specific patterns for environmental studies. BMC Genomics 15 (1), 804. doi: 10.1186/1471-2164-15-804
Li, B., Dewey, C. N. (2011). RSEM: accurate transcript quantification from RNA-seq data with or without a reference genome. BMC Bioinf. 12 (1), 323. doi: 10.1186/1471-2105-12-323
Liu, J., Milne, R. I., Zhu, G., Spicer, R. A., Wambulwa, M. C., Wu, Z., et al. (2022a). Name and scale matter: Clarifying the geography of Tibetan plateau and adjacent mountain regions. Global Planet. Change 215, 103893. doi: 10.1016/j.gloplacha.2022.103893
Liu, X. W., Wang, Y. H., Shen, S. K. (2022b). Transcriptomic and metabolomic analyses reveal the altitude adaptability and evolution of different-colored flowers in alpine Rhododendron species. Tree Physiol. 42 (5), 1100–1113. doi: 10.1093/treephys/tpab160
Lopez-Goldar, X., Agrawal, A. A. (2021). Ecological interactions, environmental gradients, and gene flow in local adaptation. Trends Plant Sci. 26 (8), 796–809. doi: 10.1016/j.tplants.2021.03.006
Love, M. I., Huber, W., Anders, S. (2014). Moderated estimation of fold change and dispersion for RNA-seq data with DESeq2. Genome Biol. 15 (12), 550. doi: 10.1186/s13059-014-0550-8
Ma, Y., Mao, X., Wang, J., Zhang, L., Jiang, Y., Geng, Y., et al. (2022b). Pervasive hybridization during evolutionary radiation of Rhododendron subgenus Hymenanthes in mountains of southwest China. Natl. Sci. Rev. 9 (12), nwac276. doi: 10.1093/nsr/nwac276
Ma, L., Sun, X., Kong, X., Galvan, J. V., Li, X., Yang, S., et al. (2015). Physiological, biochemical and proteomics analysis reveals the adaptation strategies of the alpine plant Potentilla saundersiana at altitude gradient of the northwestern Tibetan plateau. J. Proteomics 112, 63–82. doi: 10.1016/j.jprot.2014.08.009
Ma, J., Zhang, T., Wang, W., Chen, Y., Cai, W., Zhu, B., et al. (2022a). Comparative transcriptome analyses of gayal (Bos frontalis), yak (Bos grunniens), and cattle (Bos taurus) reveal the high-altitude adaptation. Front. Genet. 12. doi: 10.3389/fgene.2021.778788
Mayr, E. (1982). The growth of biological thought: Diversity, evolution, and inheritance (Cambridge, MA; London: Harvard University Press).
Miranda, V., Baker, N. R., Long, S. P. (1981). Limitations of photosynthesis in different regions of the Zea mays leaf. New Phytol. 89 (2), 179–190. doi: 10.1111/j.1469-8137.1981.tb07481.x
Mo, Z. Q., Fu, C. N., Zhu, M. S., Milne, R., Yang, J. B., Cai, J., et al. (2022). Resolution, conflict and rate shifts: insights from a densely sampled plastome phylogeny for Rhododendron (Ericaceae). Ann. Bot. 130 (5), 687–701. doi: 10.1093/aob/mcac114
Mutz, K. O., Heilkenbrinker, A., Lonne, M., Walter, J. G., Stahl, F. (2013). Transcriptome analysis using next-generation sequencing. Curr. Opin. Biotechnol. 24 (1), 22–30. doi: 10.1016/j.copbio.2012.09.004
Nagano, A. J., Kawagoe, T., Sugisaka, J., Honjo, M. N., Iwayama, K., Kudoh, H. (2019). Annual transcriptome dynamics in natural environments reveals plant seasonal adaptation. Nat. Plants 5 (1), 74–83. doi: 10.1038/s41477-018-0338-z
Naing, A. H., Kim, C. K. (2021). Abiotic stress-induced anthocyanins in plants: Their role in tolerance to abiotic stresses. Physiol. Plant 172 (3), 1711–1723. doi: 10.1111/ppl.13373
Nelson, D. R., Adger, W. N., Brown, K. (2007). Adaptation to environmental change: contributions of a resilience framework. Annu. Rev. Environ. Resour. 32 (1), 395–419. doi: 10.1146/annurev.energy.32.051807.090348
Obeso, J. R. (2002). The costs of reproduction in plants. New Phytol. 155 (3), 321–348. doi: 10.1046/j.1469-8137.2002.00477.x
Pichersky, E., Gershenzon, J. (2002). The formation and function of plant volatiles: perfumes for pollinator attraction and defense. Curr. Opin. Plant Biol. 5 (3), 237–243. doi: 10.1016/s1369-5266(02)00251-0
Qu, Y., Chen, C., Xiong, Y., She, H., Zhang, Y. E., Cheng, Y., et al. (2020). Rapid phenotypic evolution with shallow genomic differentiation during early stages of high elevation adaptation in Eurasian tree sparrows. Natl. Sci. Rev. 7 (1), 113–127. doi: 10.1093/nsr/nwz138
Raguso, R. A. (2008). Wake up and smell the roses: The ecology and evolution of floral scent. Annu. Rev. Ecol. Evol. Syst. 39, 549–569. doi: 10.1146/annurev.ecolsys.38.091206.095601
Rathee, S., Ahmad, M., Sharma, P., Singh, H. P., Batish, D. R., Kaur, S., et al. (2021). Biomass allocation and phenotypic plasticity are key elements of successful invasion of Parthenium hysterophorus at high elevation. Environ. Exp. Bot. 184, 104392. doi: 10.1016/j.envexpbot.2021.104392
Senica, M., Stampar, F., Veberic, R., Mikulic-Petkovsek, M. (2017). The higher the better? differences in phenolics and cyanogenic glycosides in Sambucus nigra leaves, flowers and berries from different altitudes. J. Sci. Food Agric. 97 (8), 2623–2632. doi: 10.1002/jsfa.8085
Shrestha, N., Wang, Z., Su, X., Xu, X., Lyu, L., Liu, Y., et al. (2018). Global patterns of Rhododendron diversity: The role of evolutionary time and diversification rates. Global Ecol. Biogeogr. 27 (8), 913–924. doi: 10.1111/geb.12750
Simao, F. A., Waterhouse, R. M., Ioannidis, P., Kriventseva, E. V., Zdobnov, E. M. (2015). BUSCO: Assessing genome assembly and annotation completeness with single-copy orthologs. Bioinformatics 31 (19), 3210–3212. doi: 10.1093/bioinformatics/btv351
Stapley, J., Reger, J., Feulner, P. G., Smadja, C., Galindo, J., Ekblom, R., et al. (2010). Adaptation genomics: the next generation. Trends Ecol. Evol. 25 (12), 705–712. doi: 10.1016/j.tree.2010.09.002
Sun, Y. B., Fu, T. T., Jin, J. Q., Murphy, R. W., Hillis, D. M., Zhang, Y. P., et al. (2018). Species groups distributed across elevational gradients reveal convergent and continuous genetic adaptation to high elevations. Proc. Natl. Acad. Sci. U.S.A. 115(45), E10634–E10641. doi: 10.1073/pnas.1813593115
Sun, H., Niu, Y., Chen, Y. S., Song, B., Liu, C. Q., Peng, D. L., et al. (2014). Survival and reproduction of plant species in the qinghai-Tibet plateau. J. Syst. Evol. 52 (3), 378–396. doi: 10.1111/jse.12092
Tanaka, Y., Sasaki, N., Ohmiya, A. (2008). Biosynthesis of plant pigments: anthocyanins, betalains and carotenoids. Plant J. 54 (4), 733–749. doi: 10.1111/j.1365-313X.2008.03447.x
Taylor, S. H., Franks, P. J., Hulme, S. P., Spriggs, E., Christin, P. A., Edwards, E. J., et al. (2012). Photosynthetic pathway and ecological adaptation explain stomatal trait diversity amongst grasses. New Phytol. 193 (2), 387–396. doi: 10.1111/j.1469-8137.2011.03935.x
Tokunaga, H., Quynh, D. T. N., Anh, N. H., Nhan, P. T., Matsui, A., Takahashi, S., et al. (2022). Field transcriptome analysis reveals a molecular mechanism for cassava-flowering in a mountainous environment in southeast Asia. Plant Mol. Biol. 109 (3), 233–248. doi: 10.1007/s11103-020-01057-0
Tong, Z. Y., Wu, L. Y., Huang, S. Q. (2021). Reproductive strategies of animal-pollinated plants on high mountains: A review of studies from the “Third pole”. J. Syst. Evol. 59 (6), 1159–1169. doi: 10.1111/jse.12680
Totland, Ø. (2001). Environment-dependent pollen limitation and selection on floral traits in an alpine species. Ecology 82 (8), 2233–2244. doi: 10.1890/0012-9658(2001)082[2233:EDPLAS]2.0.CO;2
Tu, Z., Shen, Y., Wen, S., Liu, H., Wei, L., Li, H. (2021). A tissue-specific landscape of alternative polyadenylation, lncRNAs, TFs, and gene Co-expression networks in Liriodendron chinense. Front. Plant Sci. 12. doi: 10.3389/fpls.2021.705321
Wang, X., Gao, Y., Wu, X., Wen, X., Li, D., Zhou, H., et al. (2021a). High-quality evergreen azalea genome reveals tandem duplication-facilitated low-altitude adaptability and floral scent evolution. Plant Biotechnol. J. 19 (12), 2544–2560. doi: 10.1111/pbi.13680
Wang, X., Liu, S., Zuo, H., Zheng, W., Zhang, S., Huang, Y., et al. (2021b). Genomic basis of high-altitude adaptation in Tibetan Prunus fruit trees. Curr. Biol. 31(17), 3848–3860. doi: 10.1016/j.cub.2021.06.062
Weigel, D., Nordborg, M. (2015). Population genomics for understanding adaptation in wild plant species. Annu. Rev. Genet. 49, 315–338. doi: 10.1146/annurev-genet-120213-092110
Xia, X. M., Yang, M. Q., Li, C. L., Huang, S. X., Jin, W. T., Shen, T. T., et al. (2022). Spatiotemporal evolution of the global species diversity of Rhododendron. Mol. Biol. Evol. 39 (1), msab314. doi: 10.1093/molbev/msab314
Yan, L. J., Liu, J., Moller, M., Zhang, L., Zhang, X. M., Li, D. Z., et al. (2015). DNA Barcoding of Rhododendron (Ericaceae), the largest Chinese plant genus in biodiversity hotspots of the himalaya-hengduan mountains. Mol. Ecol. Resour. 15 (4), 932–944. doi: 10.1111/1755-0998.12353
Ye, L. J., Mller, M., Luo, Y. H., Zou, J. Y., Zheng, W., Wang, Y. H., et al. (2021). Differential expressions of anthocyanin synthesis genes underlie flower color divergence in a sympatric Rhododendron sanguineum complex. BMC Plant Biol. 21 (1), 204. doi: 10.1186/s12870-021-02977-9
Ye, Z. H., Varner, J. E. (1991). Tissue-specific expression of cell wall proteins in developing soybean tissues. Plant Cell 3 (1), 23–37. doi: 10.1105/tpc.3.1.23
Yu, G., Wang, L., Han, Y., He, Q. (2012). clusterProfiler: An R package for comparing biological themes among gene clusters. OMICS: J. Integr. Biol. 16 (5), 284–287. doi: 10.1089/omi.2011.0118
Zhang, T. J., Chow, W. S., Liu, X. T., Zhang, P., Liu, N., Peng, C. L. (2016). A magic red coat on the surface of young leaves: anthocyanins distributed in trichome layer protect Castanopsis fissa leaves from photoinhibition. Tree Physiol. 36 (10), 1296–1306. doi: 10.1093/treephys/tpw080
Zhang, T., Qiao, Q., Novikova, P. Y., Wang, Q., Yue, J., Guan, Y., et al. (2019). Genome of Crucihimalaya himalaica, a close relative of Arabidopsis, shows ecological adaptation to high altitude. Proc. Natl. Acad. Sci. U.S.A. 116 (14), 7137–7146. doi: 10.1073/pnas.1817580116
Zhang, L., Xu, P. W., Cai, Y. F., Ma, L. L., Li, S. F., Li, S. F., et al. (2017). The draft genome assembly of Rhododendron delavayi franch. var. delavayi. GigaScience 6 (10), 1–11. doi: 10.1093/gigascience/gix076
Zhang, Y. Z., Xu, S. Z., Cheng, Y. W., Peng, Z. F., Han, J. M. (2018). Transcriptome profiling of anthocyaninrelated genes reveals effects of light intensity on anthocyanin biosynthesis in red leaf lettuce. PeerJ 6, e4607. doi: 10.7717/peerj.4607
Keywords: Rhododendron, elevational gradients, evolutionary adaptation, RNA-seq, organ-specific profile, alpine ecosystem
Citation: Ye L-J, Möller M, Luo Y-H, Zou J-Y, Zheng W, Liu J, Li D-Z and Gao L-M (2023) Variation in gene expression along an elevation gradient of Rhododendron sanguineum var. haemaleum assessed in a comparative transcriptomic analysis. Front. Plant Sci. 14:1133065. doi: 10.3389/fpls.2023.1133065
Received: 28 December 2022; Accepted: 06 March 2023;
Published: 21 March 2023.
Edited by:
Jian-Li Zhao, Yunnan University, ChinaReviewed by:
Jie Gao, Xishuangbanna Tropical Botanical Garden (CAS), ChinaZhiqiang Wu, Agricultural Genomics Institute at Shenzhen, CAAS, China
Copyright © 2023 Ye, Möller, Luo, Zou, Zheng, Liu, Li and Gao. This is an open-access article distributed under the terms of the Creative Commons Attribution License (CC BY). The use, distribution or reproduction in other forums is permitted, provided the original author(s) and the copyright owner(s) are credited and that the original publication in this journal is cited, in accordance with accepted academic practice. No use, distribution or reproduction is permitted which does not comply with these terms.
*Correspondence: Lian-Ming Gao, gaolm@mail.kib.ac.cn